LiftRight is a low-cost abstraction that captures upper body dynamics and computes several performance measures accurately. We leverage an arm-mounted inertial sensor for tracking the user’s arm and computing associated kinetics. We focus on segmenting the time-series workout trace from the IMU into sets which are further divided into constituent reps. For measuring user performance, LiftRight detects the range of motion and the velocity for each lifting/lowering cycle. LiftRight also determines recovery times and identifies other qualitative attributes- such as when fatigue starts setting in. This can help participants make informed decisions regarding meeting their goals. We focus on monitoring fundamental attributes for each workout. Capturing these statistics serves as a precursor to muscle fatigue detection, injury prevention, and overall health improvement.
In the real-time version of LiftRight, we use game design to merge quantifiable performance metrics with game-like elements to encourage users to perform consistent movements. Our gamified feedback approach is an exploration in understanding user needs and analyze feedback measures. We study how to enable early detection of a movement in real-time, with partial data. To generate actionable information via early event detectionin real-world conditions we define the following design goals: scalable, translatable, unobtrusive, and real-time. We believe that the ability to accurately monitor weight training will lower the entrance barrier and help prevent injuries by helping users and trainers alike.
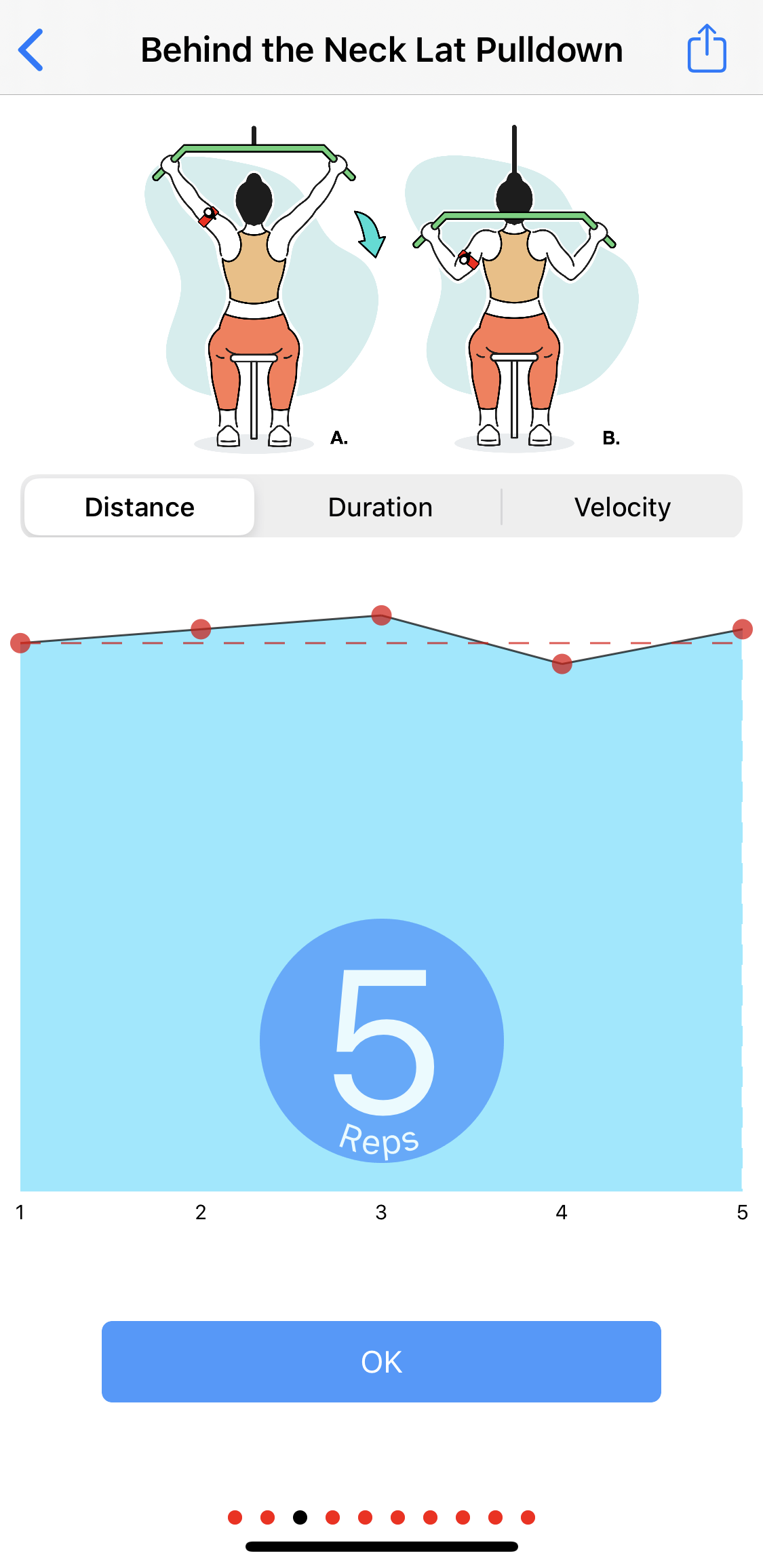